Detecting esophageal cancer using surface-enhanced Raman spectroscopy: a narrative review
Introduction
Esophageal cancer is the 5th most common gastrointestinal cancer in the U.S. (1). Esophageal adenocarcinoma incidence rates have been rising and patients often present with metastasis due to minimal early stage symptoms, contributing to an overall poor 5-year survival rate of about 20% (1-4). As such, early detection and initiation of treatment is necessary to improve long-term survival rates of esophageal cancer (5). The current gold standard for diagnosis is endoscopic biopsy. However, endoscopic visualizations and biopsies are invasive, time-consuming, and operator dependent, and are often conducted late in the disease course. Therefore, it is important to develop alternative methods for detecting esophageal cancer that are non-invasive, sensitive, and can provide ongoing disease surveillance.
For this purpose, surface-enhanced Raman spectroscopy (SERS) has been of increasing interest. Raman spectroscopy (RS) is a technique that uses frequency shifts of incident light on materials to generate Raman spectra unique to each substrate that can be used to accurately determine material compositions. SERS amplifies RS using roughened surfaces and/or nanoparticles (NPs) made of gold or silver to increase RS signal intensity. SERS can be applied to tissues, cells, serum, urine, and many other biological samples for both diagnostic and therapeutic purposes. Many studies have combined SERS with machine learning algorithms to accurately distinguish between cancerous and noncancerous samples, potentially changing the diagnosis, monitoring, and management of cancer (6-9). Here, we explore current advancements in the utility of SERS for esophageal cancers and its potential for future clinical translation. We present the following article in accordance with the Narrative Review reporting checklist (available at https://aoe.amegroups.com/article/view/10.21037/aoe-22-24/rc).
Methods
Studies on SERS for esophageal cancer application were identified by searching the following databases: Web of Science (all databases), PubMed, Google Scholar, and PLOS One (all databases). Keywords used included: “Raman spectroscopy”, “esophageal cancer”, “esophageal neoplasms”, “surface-enhanced Raman spectroscopy”, “SERS”, and “nanoparticles”. Only relevant literature on SERS for esophageal cancer detection was included in this study. The search strategy is summarized in Table 1. We identified 12 articles that used SERS-based technology for esophageal cancer detection from our literature search. These articles are summarized in Table 2.
Table 1
Items | Specification |
---|---|
Date of search | January 1st 2021–December 31st 2021 |
Databases and other sources searched | Web of Science (all databases), PubMed, Google Scholar, and PLOS One (all databases) |
Search terms used | “Raman spectroscopy”, “esophageal cancer”, “esophageal neoplasms”, “surface-enhanced Raman spectroscopy”, “SERS”, and “nanoparticles” |
Timeframe | January 1st 2010–December 31st 2021 |
Inclusion and exclusion criteria | Studies meeting search criteria and relevant to literature on SERS for esophageal cancer detection were included in this study. Only English studies were included |
Selection process | Selection of articles was conducted independently by 2 separate investigators (SH, DN) and disagreements were resolved through discussion to generate a single consensus list |
Table 2
Author, date | Study type, study model | Nanoparticle design | Sample medium | Sample population characteristics | Raman parameters (laser wavelength, Raman shift range) | Statistical analysis | Key Raman peaks and valleys (cm−1)† | Diagnostic accuracy (sensitivity, specificity) |
---|---|---|---|---|---|---|---|---|
Li, 2014 (10) | In vitro, human | Ag NPs | Blood plasma | 36 esophageal cancer patients, 50 healthy patients | 785 nm, 400–1,800 cm−1 | PCA-LDA, SVM | Peaks: 725, 1,333, 1,400, 1,578 | PCA-LDA: 94.4%, 100% |
Valleys: 494, 589, 636, 811, 886, 1,064, 1,133 | SVM: 86.1%, 100% | |||||||
Huang, 2014 (11) | In vitro, human | Ag NPs | Urine | 56 esophageal cancer patients, 36 healthy patients | 785 nm, 400–1,800 cm−1 | PCA-LDA | Peaks: 725, 1,465 | PCA-LDA: 89.3%, 83.3% |
Valleys: 527, 889, 1,138, 1,002 | ||||||||
Li, 2015 (6) | In vitro, human | Ag NPs | Blood serum | 22 esophageal cancer patients, 23 healthy patients | 632.8 nm, 400–1,800 cm−1 | PCA, HCA | Peaks: 495, 1,132, 1,323, 1,355 | PCA: 90.9%, 91.3% |
Valleys: 636, 727, 886 | HCA: 77.3%, 65.2% | |||||||
Zhou, 2014 (12) | In vitro, human | Ag NPs | Blood hemoglobin | 30 esophageal cancer patients, 30 healthy patients | 532 nm, 600–1,800 cm−1 | PCA-DA | Valleys: 1,562, 1,591, 1,639 | PCA-DA: 86.7%, 93.3% |
Feng, 2017 (13) | In vitro, human | Au NPs | Urine | 55 esophageal cancer patients, 52 healthy patients | 785 nm, 600–1,700 cm−1 | PLS-DA | Peaks: 765, 1,184 | PLS-DA: 100%, 100% |
Valleys: 725, 863, 1,475 | ||||||||
Li, 2013 (14) | In vitro, human | Ag NPs | Blood serum | 30 esophageal cancer patients, 31 healthy patients | 785 nm, 600–1,800 cm−1 | PCA-SVM, C-SVM | Valleys: 1,219, 1,445, 1,585 | PCA-SVM: 83.3%, 86.7% |
PCA-LDA: 76.7%, 77.4% | ||||||||
Wang, 2015 (15) | In vivo, rat | Au NPs | Tissue | 3 tumor explants | 785 nm, 700–2,000 cm−1 | Spectral demultiplexing | – | – |
Zavaleta, 2013 (16) | In vitro, human | Au NPs | Tissue | 3 healthy patients§ | 785 nm, 700–2,000 cm−1 | – | – | – |
Feng, 2013 (7) | In vitro, human | Ag NPs | Tissue | 44 esophageal cancer patients‡ | 785 nm, 300–1,800 cm−1 | PCA-LDA | Peaks: 478, 1,211, 1,583 | PCA-LDA: 90.9%, 97.8% |
Valleys: 653, 725, 1,375 | ||||||||
Wang, 2013 (17) | In vitro, human | Au NPs | Tissue | 31 esophageal cancer patients¶ | 785 nm, 400–1,750 cm−1 | PCA-LDA | Peaks: 1,246, 1,587 | PCA-LDA: 90.3%, 90.3% |
Valleys: 621, 643, 1,090, 1,367, 1,454 | ||||||||
Liu, 2014 (18) | In vitro, human | Au NPs | Cell suspension | – | 785 nm, 200–1,800 cm−1 | – | – | – |
Harmsen, 2019 (19) | In vivo, mouse | Au NPs | Tissue | 5 esophageal cancer model mice |
785 nm, 500–1,500 cm−1 | – | – | – |
†, peaks and valleys of esophageal cancer sample spectra in comparison to healthy sample spectra. §, the pilot endoscope was tested on 3 healthy patients without NP administration. NP multiplexing capacity was tested on human and pig colon tissue. ‡, both healthy and esophageal cancer tissue samples were collected from 44 esophageal cancer patients, generating a total of 44 esophageal cancer tissue samples and 44 healthy tissue samples. ¶, both healthy and esophageal cancer tissue samples were collected from 31 esophageal cancer patients, generating a total of 31 esophageal cancer tissue samples and 31 healthy tissue samples. SERS, surface-enhanced Raman spectroscopy; NP, nanoparticle; PCA, principal component analysis; LDA, linear discriminant analysis; SVM, support vector machine; HCA, hierarchical cluster analysis; PLS-DA, partial least squares discriminant analysis.
Narrative
SERS: overview and parameters
Classic Raman scattering was described by C. V. Raman in 1928, who found that inelastic collisions between incident photons and molecules caused a documentable shift in photon frequency (20). These shifts are characteristic of molecular bond vibrations caused by photon collisions, generating unique “Raman spectra” for individual molecules. This spurred a great deal of interest in utilizing RS for chemical analysis. In particular, biomolecular Raman peaks have been extensively documented as researchers attempted to use RS for analytical and diagnostic applications. Its ability to collect unique spectra from a variety of substrates, combined with a relatively straightforward experimental setup (via laser excitation), makes RS a highly attractive tool (21). However, a major drawback of RS is that its signal is relatively weak. Combined with other limitations such as low detection ability, fluorescent interference and long exposure times to potentially harmful lasers, RS still had distinct areas for improvement (22).
SERS improves upon RS by dramatically increasing the Raman signal by orders of magnitude of at least 103, and in certain studies, by 1014–1016 (23,24). This is through the use of novel nanotechnology platforms which contain a metal, such as gold or silver, that is contused with a Raman reporter shell. It is theorized that electromagnetic and chemical mechanisms both contribute to this enhancement (Figure 1). First, electromagnetic enhancement is believed to contribute to the majority of signal amplification and occurs when incident light causes oscillation of valence electrons in local atoms when the light’s frequency matches the electrons’ resonant frequency (a phenomenon called local surface plasmon resonance). Enhancement can then be considered to occur in a two-step process: first locally onto the substrate, and then as the substrate enhances the emitted field from the analyte. In this way, emitted fields from the analyte of interest can be considered “re-emitted” from the substrate (Figure 1A) (25). This process occurs particularly at “hot spots” where two NPs are in close proximity to each other. Modifications to SERS substrate structures are therefore sought to promote “hot spots” and enhance the Raman signal (24,26,27). The second mechanism, chemical enhancement, is less well understood and is believed to contribute less towards signal amplification. It is thought that one aspect of chemical enhancement occurs when new complexes are formed between the analyte and the substrate; this new complex subsequently enhances the Raman signal (Figure 1B). Because chemical enhancement depends in part on the analyte, this may explain why certain molecules are not effective with the SERS technique or why SERS enhancement factors among different analytes vary with the same SERS substrate (27-30). Today, a variety of SERS techniques ranging from roughening surfaces to noble metal nanostructures are used.
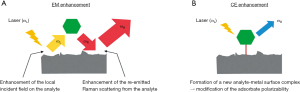
SERS methodology and statistical analysis
Methodological considerations in biological SERS studies include the choice of substrate, sample medium, instrumentation, and statistical analysis. In regard to SERS substrates, colloidal NPs are commonly used as SERS-active substrates for their ease of synthesis, tailored surface properties, and ability to enhance Raman signals on a variety of samples. Silver and gold are commonly-utilized noble metals for colloidal NPs because they exhibit localized surface plasmon resonance in the visible and near-infrared range, conjugate well to surface modifications, and resist corrosion (24,26,31). All the studies reported here utilize silver or gold NPs (Table 2). Two studies report using a simple deoxidizing method developed by Leopold and Lendl to generate Ag colloids, whereupon silver nitrate is reduced by hydroxylamine hydrochloride to generate Ag NPs (10,11,32). Reduction with sodium citrate is another commonly reported method of synthesis (6). NP specificity is further improved through tailored surface modifications such as antibodies and peptides specific to the target biomolecule. For example, NPs conjugated by antibodies specific to epidermal growth factor receptor (EGFR) (expressed preferentially in esophageal cancer cells) allow NPs to hone to cancer sites. This is assisted by Raman reporter molecules, which can also be conjugated to NPs and provide a distinct peak to indicate the presence of the target. Together, surface modifications make Raman NPs especially useful for multiplexed imaging purposes (31,33). Colloidal NP solutions have versatile applications and can be mixed with patient sample mediums for direct SERS imaging, applied to tissue sections, or even incorporated into fiber optic sensors (Table 2). Sample mediums therefore vary widely and can be either fluid or solid. Fluid sample mediums include patient urine, blood hemoglobin, and blood plasma; solid sample mediums include tissue biopsies or endoscopy of the esophagus (Table 2).
SERS methodology does not vary significantly from RS instrumentation and has been previously discussed (34). In brief, a laser with an excitation wavelength near the resonance frequency of the sample is directed at the sample. For example, one study reports using a 632.8 nm laser excitation wavelength because the SERS-active substrate exhibited a maximum absorption wavelength of 418 nm (12). However, the majority of SERS-based esophageal cancer studies reported here use a 785 nm laser excitation wavelength, which has previously been reported to minimize background fluorescence and maximize signal sensitivity (35,36). Biological samples emit signals at various wavelengths, so a wide collection range is necessary in order to collect the full range of signals emitted by samples (21). All of the studies reported here collected spectroscopy signals within the range of 300–2,000 nm (Table 2).
Principal component analysis (PCA) and discriminant analysis (DA) are two statistical methods commonly employed for SERS data. PCA and DA reduce dimensionality of the data to maximally account for variance with the minimum number of variables. The main difference between PCA and DA is that PCA is an unsupervised method that does not account for group labels (e.g., cancerous vs. healthy samples), whereas DA is a supervised method that uses pre-given group labels to maximize the difference between groups (37,38). This means that while DA is a categorization that distinguishes patients with cancerous SERS spectra from healthy patients’ SERS spectra, PCA only reduces dimensionality. For this reason, PCA is mostly used in conjunction with DA in SERS esophageal cancer data (Table 2). Other methods reported include hierarchical cluster analysis (HCA, unsupervised), support vector machine (SVM, supervised), and partial least squares (PLS) analysis, all of which similarly operate to categorize data. The ability of these algorithms to distinguish between cancerous and healthy samples is reported as its diagnostic sensitivity and specificity (Table 2).
SERS analysis by sample medium
Key differences have been found in prior studies between healthy and esophageal cancer patients’ SERS spectra (6,7,10-19). Because SERS spectra and results vary by the sample medium, herein we describe SERS results grouped by sample medium.
Urine SERS spectra
Urine SERS analysis is performed by mixing colloidal NPs with urine samples and has been conducted by several studies with varying success. Huang et al. reported using urine samples from 56 esophageal cancer patients and 36 healthy patients to distinguish between healthy and cancer patients with 89.3% sensitivity and 83.3% specificity (11). Key differences between healthy and cancer patients were found in SERS peaks. Tentative signal assignment found that both uric acid (725 cm−1) and tryptophan (1,465 cm−1) were increased in cancer patients, whereas nucleic acids (527 cm−1), D-galactosamine (889 cm−1), urea (1,002 cm−1), and phenylalanine (1,138 cm−1) were decreased in cancer patients (Figure 2) (11). These results suggest increased DNA breakage and differences in amino acid/polysaccharide metabolism in esophageal cancer patients (11). Another study by Feng et al. used urine samples and was able to distinguish between healthy and cancer patients with 100% sensitivity and 100% specificity (13). One reason for the discrepancy in detection may be methodology; rather than directly mixing NPs with patient urine samples, Feng et al. isolated modified nucleosides from patient urine using affinity chromatography prior to analysis with gold colloidal solution. They also utilized PCA-DA analysis rather than PCA-LDA analysis. Feng et al. found lower peak signals assigned to adenine vibration (725 cm−1) in cancer patients, which the authors suggest indicates altered RNA metabolism in esophageal cancer patients (13). Interestingly, both studies identified 725 cm−1 as a key peak difference between esophageal cancer patients and healthy patients. Huang et al. interpreted 725 cm−1 to suggest higher uric acid content in cancer samples, whereas Feng et al. interpreted 725 cm−1 to suggest lower adenine content in cancer samples. This demonstrates how different signal assignments can result in vastly different interpretations. This may also be related to methodology, as the latter study isolated modified nucleosides and likely had insignificant amounts of uric acid in solution.
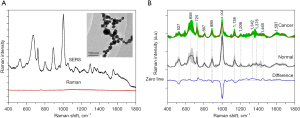
Blood SERS spectra
Blood SERS studies are conducted by mixing blood components with colloidal NPs. Several studies used SERS to analyze blood serum, plasma, and isolated hemoglobin for esophageal cancer detection. Using blood serum samples, a study by Li et al. distinguished between 31 healthy patients and 30 cancer patients’ sera with 83.3% sensitivity and 86.7% specificity (14). Cancer patients had markedly lower peaks in signals assigned to collagen/lipids (1,445 cm−1), suggesting lower fat content in esophageal cancer patients’ sera due to increased fat consumption by cancer cells. Cancer patients had higher peaks in signals assigned to β-helix proteins (1,620 cm−1–1,670 cm−1), suggesting increased presence of β-helix proteins in their sera. Another study by Li et al. distinguished between healthy and cancer patients’ sera with 90.9% sensitivity and 91.3% specificity (6). This study similarly found increased peaks in signals tentatively assigned to amino acids such as tryptophan (1,355 cm−1) and arginine (495 cm−1), in addition to increased peaks in signals assigned to nucleotides (1,355 cm−1). These findings suggest that abnormal protein and nucleotide metabolism in esophageal cancer patients is discernable by SERS. In addition, the study found a decreased peak in another signal assigned to tryptophan (886 cm−1). This discrepancy between increased and decreased tryptophan levels demonstrates the uncertainty when assigning peaks to biomolecules. Inter-study discrepancies also exist; the latter study found higher peaks in signals assigned to collagen/tryptophan (1,323 cm−1), contradicting the former study’s finding of decreased collagen metabolism in cancer patients. A possible cause for the discrepancy between studies could be different assignment of signals, as the former and latter studies assigned different signal shifts to collagen (1,445 cm−1 and 1,323 cm−1, respectively).
Apart from blood serum, we identified SERS studies that were conducted using blood plasma and hemoglobin. Li et al. used blood plasma to distinguish between cancer and healthy patient samples with 94.4% sensitivity and 100% specificity (10). Cancer patients had increased peaks in signals assigned to nucleotides (725 cm−1, adenine; 1,333 cm−1, nucleic acid bases). This finding supports several other studies and suggests that abnormal DNA and RNA metabolism frequently distinguishes cancer patients’ blood from healthy patients’ blood. However, it is in contrast to Feng et al.’s study, which found a lower peak at 725 cm−1 (adenine) rather than an increased peak at that signal. Feng et al. suggested this indicated abnormal RNA metabolism, and the discrepancy may be due to different sample mediums or methodology (13). Interestingly, a study by Zhou et al. using hemoglobin found that cancer patients had more low spin state iron ions in their hemoglobin compared to healthy patients. Because iron ions are at a low spin state when combined with oxygen, the authors argue that this indicates greater hemolysis and free iron combined with oxygen in cancer patients (12).
Tissue
Tissue-based SERS analysis relies upon directly applying NPs to target tissue. NPs are designed to have affinity for cancerous surface markers or overexpressed proteins; in a patient with esophageal carcinoma, these NPs are designed to bind to literature-confirmed overexpressed proteins such as EGFR and human epidermal growth factor receptor (HER2) (15). For in vivo studies, NPs are consumed similar to a barium swallow. A fiber optic probe is then inserted into the esophagus to directly visualize esophageal tissue coated with NPs and used to generate spectra. Several studies have demonstrated the efficacy of this method. One recent study implanted esophageal carcinoma tumor xenografts in rodent esophagi and demonstrated unique SERS peaks at wavelengths consistent with molecules involved in carcinogenesis, such as the aforementioned EGFR and HER2 (15). Another study developed a flexible fiber optic probe for endoscopy. This probe was piloted on 3 patients during their routine colonoscopies, although no NPs were administered to the patients prior to using the probe. The probe was also tested on human colon tissue biopsies with multiple types of gold NPs applied. The probe distinguished between different NPs with 100% sensitivity and 89% specificity, demonstrating its potential for future clinical usage (16).
For in vitro studies, NPs are directly applied to a tissue biopsy and visualized with SERS. For example, one study used 44 excised esophageal tissue samples two hours post-resection from cancer patients, along with 44 controls (7). This study found unique and clear SERS peaks at wavelengths also consistent with carcinogenesis. For example, esophageal cancer samples had higher peaks in signals assigned to exocyclic thymine deformation bands (478 cm−1), which is consistent with a past SERS study on nasopharyngeal carcinoma (8). Cancer samples also had higher peaks in signals assigned to phenylalanine (1,211 cm−1), consistent with SERS literature showing this amino acid to be overexpressed in malignancy (39). Principal discriminant analysis-linear discriminant analysis (PDA-LDA) distinguished between cancerous and esophageal samples with nearly 91% sensitivity and 98% specificity.
Discussion
Esophageal cancer has one of the poorest 5-year survival rates in large part because patients frequently present with advanced disease. This makes early diagnosis and monitoring of esophageal cancer crucial, particularly for populations like those with Barrett’s esophagus, gastroesophageal reflux disease, and smokers. As such, detection of esophageal cancer using SERS is promising for its noninvasive sampling technique and high diagnostic accuracy. Urine and blood analysis are routinely conducted in the clinic, making SERS translatable for patient diagnosis and ongoing patient monitoring. Moreover, they may be used for disease monitoring following treatment to monitor for recurrence. Fiber optic SERS applied in vivo are also promising for their ability to be administered alongside routine endoscopies. However, an important consideration in tissue-based SERS is the clinical optimization of the Raman endoscopes. Numerous studies have used endoscopes that utilize lasers that surpass the American National Standards Institute’s suggested maximum permissible exposure and may cause burning or other adverse effects when used in practice (7,15,16). To this end, Zavaleta et al. used unique fiber optic scanners that use acceptable laser powers, while attempting to maintain high levels of sensitivity and specificity demonstrated in other trials (16).
The results reported by studies indicate that SERS-based machine learning algorithms have great promise for esophageal cancer detection. Prior studies have found that histologic diagnosis of esophageal cancer biopsies can be near 70−90% sensitivity and specificity (40-42). While biopsies remain the gold standard for diagnosis, the high sensitivities and specificities of SERS algorithms demonstrate their potential for disease screening and monitoring. PCA, DA and other techniques are being employed with increasing frequency for clinical applications, from prostate cancer detection to radiographic image analysis, and so the usage of such algorithms also demonstrates the applicability of machine learning techniques to esophageal cancer detection. Furthermore, diagnostic accuracy of principal components analysis/discriminant analysis (PCA/DA) algorithms reflects both the algorithm’s accuracy and the degree of difference between cancer and healthy patients’ SERS spectra. Recent advancements in identifying key cancer biomarkers may be helpful by “widening the gap” between healthy and cancerous samples and thereby further refine algorithms.
Limitations of SERS for esophageal cancer detection include inconsistent assignment of signals, limited diffusion depth, and limited data for algorithm training. Because signals are tentatively assigned to possible biomolecules, studies may conflict in their spectra interpretations if they assign different signals to the same biomolecule. This results in inconsistent conclusions that make comparisons between SERS studies difficult. Application of SERS for multiplexed imaging purposes is also limited by its poor diffusion depth compared to MRI or CT. Recent advances in NP synthesis have sought to optimize signal intensity by optimizing NP shapes, sizes, and materials; for example, SERS gold nanostars generate improved “hot spots” that amplify the SERS signal (43). In addition, like all machine learning algorithms, the diagnostic accuracy of PCA and DA SERS-based algorithms increases with more samples. Limited or biased samples can skew algorithms towards faulty categorizations that impede the ability to detect patients with esophageal cancer. As such, future studies should further optimize their results by using larger sample sizes. SERS is still an early-stage technology that has limited clinical validation, and human in vivo studies are needed to validate SERS for clinical use. Limitations of this review include a small sample of included studies and significant heterogeneity in methodology and results between studies. Cross-collaboration between research groups is necessary to standardize methodologies for consistent results.
Conclusions
Detection of esophageal cancer using SERS is an early-stage but promising tool due to its high diagnostic accuracy and noninvasive sampling technique. Various studies have employed SERS on urine, blood, and tissue components to generate Raman spectra. These spectra revealed multiple differences between cancerous and healthy samples, and signals tentatively assigned to peaks/valleys suggest findings such as abnormal DNA/RNA metabolism and abnormal amino acid metabolism. Machine learning techniques such as PCA and DA distinguished between esophageal cancer and healthy patients’ spectra with high sensitivity and specificity. Future studies are needed to standardize SERS methodology and validate SERS in vivo for human use.
Acknowledgments
Funding: None.
Footnote
Reporting Checklist: The authors have completed the Narrative Review reporting checklist. Available at https://aoe.amegroups.com/article/view/10.21037/aoe-22-24/rc
Peer Review File: Available at https://aoe.amegroups.com/article/view/10.21037/aoe-22-24/prf
Conflicts of Interest: All authors have completed the ICMJE uniform disclosure form (available at https://aoe.amegroups.com/article/view/10.21037/aoe-22-24/coif). The authors have no conflicts of interest to declare.
Ethical Statement:
Open Access Statement: This is an Open Access article distributed in accordance with the Creative Commons Attribution-NonCommercial-NoDerivs 4.0 International License (CC BY-NC-ND 4.0), which permits the non-commercial replication and distribution of the article with the strict proviso that no changes or edits are made and the original work is properly cited (including links to both the formal publication through the relevant DOI and the license). See: https://creativecommons.org/licenses/by-nc-nd/4.0/.
References
- Mukkamalla SKR, Recio-Boiles A, Babiker HM. Esophageal Cancer. Treasure Island (FL): StatPearls Publishing; 2022.
- SEER. SEER Cancer Statistics Review (CSR) 1975-2016. [cited 2022 Mar 1]. Available online: https://seer.cancer.gov/archive/csr/1975_2016/
- Halpern AL, McCarter MD. Palliative Management of Gastric and Esophageal Cancer. Surg Clin North Am 2019;99:555-69. [Crossref] [PubMed]
- Njei B, McCarty TR, Birk JW. Trends in esophageal cancer survival in United States adults from 1973 to 2009: A SEER database analysis. J Gastroenterol Hepatol 2016;31:1141-6. [Crossref] [PubMed]
- Abbas G, Krasna M. Overview of esophageal cancer. Ann Cardiothorac Surg 2017;6:131-6. [Crossref] [PubMed]
- Li X, Yang T, Li S, et al. Detecting Esophageal Cancer Using Surface-Enhanced Raman Spectroscopy (SERS) of Serum Coupled with Hierarchical Cluster Analysis and Principal Component Analysis. Appl Spectrosc 2015;69:1334-41. [Crossref] [PubMed]
- Feng S, Lin J, Huang Z, et al. Esophageal cancer detection based on tissue surface-enhanced Raman spectroscopy and multivariate analysis. Appl Phys Lett 2013;102:043702. [Crossref]
- Feng S, Chen R, Lin J, et al. Nasopharyngeal cancer detection based on blood plasma surface-enhanced Raman spectroscopy and multivariate analysis. Biosens Bioelectron 2010;25:2414-9. [Crossref] [PubMed]
- Abedi V, Li J, Shivakumar MK, et al. Increasing the Density of Laboratory Measures for Machine Learning Applications. J Clin Med 2020;10:103. [Crossref] [PubMed]
- Li D, Feng S, Huang H, et al. Label-free detection of blood plasma using silver nanoparticle based surface-enhanced Raman spectroscopy for esophageal cancer screening. J Biomed Nanotechnol 2014;10:478-84. [Crossref] [PubMed]
- Huang S, Wang L, Chen W, et al. Potential of non-invasive esophagus cancer detection based on urine surface-enhanced Raman spectroscopy. Laser Phys Lett 2014;11:115604. [Crossref]
- Zhou X, Diao Z, Fan C, et al. Surface-enhanced Raman spectra of hemoglobin for esophageal cancer diagnosis. Eur Phys J Appl Phys 2014;65:30701. [Crossref]
- Feng S, Zheng Z, Xu Y, et al. A noninvasive cancer detection strategy based on gold nanoparticle surface-enhanced raman spectroscopy of urinary modified nucleosides isolated by affinity chromatography. Biosens Bioelectron 2017;91:616-22. [Crossref] [PubMed]
- Li SX, Zeng QY, Li LF, et al. Study of support vector machine and serum surface-enhanced Raman spectroscopy for noninvasive esophageal cancer detection. J Biomed Opt 2013;18:27008. [Crossref] [PubMed]
- Wang YW, Kang S, Khan A, et al. In vivo multiplexed molecular imaging of esophageal cancer via spectral endoscopy of topically applied SERS nanoparticles. Biomed Opt Express 2015;6:3714-23. [Crossref] [PubMed]
- Zavaleta CL, Garai E, Liu JT, et al. A Raman-based endoscopic strategy for multiplexed molecular imaging. Proc Natl Acad Sci U S A 2013;110:E2288-97. [Crossref] [PubMed]
- Wang Y, Chen W, Huang Z, et al. Diagnosis of Esophageal Tissue Based on Surface-Enhanced Raman Spectroscopy. Sci Sin Vitae 2013;43:525-32. [Crossref]
- Liu S, Li L, Chen Z, et al. Surface-enhanced Raman spectroscopy measurement of cancerous cells with optical fiber sensor. Chinese Optics Letters 2014;12:S13001. [Crossref]
- Harmsen S, Rogalla S, Huang R, et al. Detection of Premalignant Gastrointestinal Lesions Using Surface-Enhanced Resonance Raman Scattering-Nanoparticle Endoscopy. ACS Nano 2019;13:1354-64. [Crossref] [PubMed]
- Singh R C. V.. Raman and the Discovery of the Raman Effect. Phys Perspect 2002;4:399-420. [Crossref]
- Movasaghi Z, Rehman S, Rehman IU. Raman spectroscopy of biological tissues. Appl Spectrosc Rev 2007;42:493-541. [Crossref]
- Araujo CF, Nolasco MM, Ribeiro AMP, et al. Identification of microplastics using Raman spectroscopy: Latest developments and future prospects. Water Res 2018;142:426-40. [Crossref] [PubMed]
- Samanta A, Maiti KK, Soh KS, et al. Ultrasensitive near-infrared Raman reporters for SERS-based in vivo cancer detection. Angew Chem Int Ed Engl 2011;50:6089-92. [Crossref] [PubMed]
- Stiles PL, Dieringer JA, Shah NC, et al. Surface-enhanced Raman spectroscopy. Annu Rev Anal Chem (Palo Alto Calif) 2008;1:601-26. [Crossref] [PubMed]
- Guerrini L, Graham D. Molecularly-mediated assemblies of plasmonic nanoparticles for Surface-Enhanced Raman Spectroscopy applications. Chem Soc Rev 2012;41:7085-107. [Crossref] [PubMed]
- Zong C, Xu M, Xu LJ, et al. Surface-Enhanced Raman Spectroscopy for Bioanalysis: Reliability and Challenges. Chem Rev 2018;118:4946-80. [Crossref] [PubMed]
- Haynes CL, McFarland AD, Van Duyne RP. Surface-enhanced: Raman spectroscopy. Anal Chem 2005;77:338 A-346 A.
- Moskovits M. Surface-enhanced Raman spectroscopy: a brief retrospective. J Raman Spectrosc 2005;36:485-96. [Crossref]
- Kneipp K, Kneipp H, Itzkan I, et al. Surface-enhanced Raman scattering and biophysics. J Phys Condens Matter 2002;14:R597. [Crossref]
- Langer J, Jimenez de Aberasturi D, Aizpurua J, et al. Present and Future of Surface-Enhanced Raman Scattering. ACS Nano 2020;14:28-117. [Crossref] [PubMed]
- Sánchez-Purrà M, Roig-Solvas B, Rodriguez-Quijada C, et al. Reporter Selection for Nanotags in Multiplexed Surface Enhanced Raman Spectroscopy Assays. ACS Omega 2018;3:10733-42. [Crossref] [PubMed]
- Leopold N, Lendl B. A New Method for Fast Preparation of Highly Surface-Enhanced Raman Scattering (SERS) Active Silver Colloids at Room Temperature by Reduction of Silver Nitrate with Hydroxylamine Hydrochloride. J Phys Chem B 2003;107:5723-7. [Crossref]
- Smolsky J, Kaur S, Hayashi C, et al. Surface-Enhanced Raman Scattering-Based Immunoassay Technologies for Detection of Disease Biomarkers. Biosensors (Basel) 2017;7:7. [Crossref] [PubMed]
- Hiremath G, Locke A, Sivakumar A, et al. Clinical translational application of Raman spectroscopy to advance Benchside biochemical characterization to bedside diagnosis of esophageal diseases. J Gastroenterol Hepatol 2019;34:1911-21. [Crossref] [PubMed]
- Yuan H, Fales AM, Khoury CG, et al. Spectral Characterization and Intracellular Detection of Surface-Enhanced Raman Scattering (SERS)-Encoded Plasmonic Gold Nanostars. J Raman Spectrosc 2013;44:234-9. [Crossref] [PubMed]
- Wang P, Zhang D, Zhang L, Fang Y. The SERS study of graphene deposited by gold nanoparticles with 785 nm excitation. Chem Phys Lett 2013;556:146-50. [Crossref]
- Brown MT, Wicker LR. Discriminant Analysis. In: Tinsley H, Brown S. editors. Handbook of Applied Multivariate Statistics and Mathematical Modeling. 1st ed. Elsevier; 2000:209-35.
- Ivosev G, Burton L, Bonner R. Dimensionality reduction and visualization in principal component analysis. Anal Chem 2008;80:4933-44. [Crossref] [PubMed]
- Huang Z, McWilliams A, Lui H, et al. Near-infrared Raman spectroscopy for optical diagnosis of lung cancer. Int J Cancer 2003;107:1047-52. [Crossref] [PubMed]
- Nagai K, Ishihara R, Ishiguro S, et al. Endoscopic optical diagnosis provides high diagnostic accuracy of esophageal squamous cell carcinoma. BMC Gastroenterol 2014;14:141. [Crossref] [PubMed]
- Muto M, Minashi K, Yano T, et al. Early detection of superficial squamous cell carcinoma in the head and neck region and esophagus by narrow band imaging: a multicenter randomized controlled trial. J Clin Oncol 2010;28:1566-72. [Crossref] [PubMed]
- Yoshida T, Inoue H, Usui S, et al. Narrow-band imaging system with magnifying endoscopy for superficial esophageal lesions. Gastrointest Endosc 2004;59:288-95. [Crossref] [PubMed]
- Song C, Li F, Guo X, et al. Gold nanostars for cancer cell-targeted SERS-imaging and NIR light-triggered plasmonic photothermal therapy (PPTT) in the first and second biological windows. J Mater Chem B 2019;7:2001-8. [Crossref] [PubMed]
Cite this article as: Hu S, Brooks D, Nemirovsky D, Gupta P, Chiu B, Razavi M. Detecting esophageal cancer using surface-enhanced Raman spectroscopy: a narrative review. Ann Esophagus 2024;7:4.