Development of artificial intelligence for the detection and staging of esophageal cancer
Introduction
In recent years, computer-assisted diagnosis (CAD) using machine learning systems has received significant attention and made remarkable progress. Deep learning, a subset of machine learning, involves various machine learning models, usually based on convolutional neural networks (CNNs). The difference between classic machine learning and CNNs is that the former is based on manually selected features, such as color, texture, and shape, intended to imitate an endoscopist’s analysis, whereas the latter is an end-to-end learning system with automatic feature learning (1). Because the computer automatically performs feature selection, understanding the outcome is challenging, and thus, CNN is often referred to as a “black box” (2). CNN has achieved expert capabilities in multiple medical fields, including radiology, skin lesions, retinal screening, and pathology slides (3-5).
The application of the CAD system to diagnose early esophageal squamous cell carcinoma (ESCC) has also received attention in recent times. In endoscopic applications, the CAD system was first used in colonoscopies to help improve polyp detection and adenoma detection and to interpret the lesion patterns, differentiating between benign and precancerous polyps (6). However, the endoscopic diagnosis of esophageal cancer is not objective; it relies on the skills and experience of the endoscopist (7,8), and accurate diagnosis requires significant experience and time. Rodríguez de Santiago et al. reported that less experienced endoscopists (<5 years and <1,000 endoscopies) were strongly associated with missed esophageal cancer on multivariate analysis (8). The application of a CAD system has the potential to solve both the difficulty and complexity of endoscopic diagnosis. With such systems, all endoscopists, including non-experts, could easily achieve accurate diagnoses.
This article reviews the current status of artificial intelligence (AI)-driven CAD systems in the detection and staging of early stage esophageal cancer.
Prevalence of esophageal cancer
Esophageal cancer is one of the leading causes of cancer-related death and is associated with high morbidity and mortality (9,10). There are two predominant histologic types of esophageal cancer: ESCC and esophageal adenocarcinoma (EAC) in Barrett’s esophagus (BE). Although EAC is predominant in Western countries, ESCC is still the most common subtype, accounting for 80% of all esophageal cancers (11).
Detection of squamous cell carcinoma
Although the overall prognosis of patients with advanced ESCC is poor, it can be treated in a less-invasive manner, for example, by endoscopic resection, if the lesion is detected at an early (mucosal or submucosal) stage (12-17). Therefore, detecting the lesions in their early stages is very important. However, it has been a great challenge to diagnose ESCC at such an early stage through conventional endoscopy, particularly using white light imaging (WLI) (18,19). Iodine staining is useful and widely used to detect ESCC in high risk patients; however, it is linked to chest discomfort. In contrast, NBI and blue laser imaging (BLI) are revolutionary image-enhanced endoscopy technologies that make superficial ESCC detection easy (20-26). NBI is superior to iodine staining owing to its simplicity in operation; it can be performed within minutes by pushing a single button, causing no discomfort to the patient. However, when used by less experienced endoscopists, its sensitivity is only 53% for detecting ESCC (27). Therefore, there is a need for novel, effective technologies to effectively detect superficial ESCC.
Horie et al. first reported an AI-driven CAD system for detecting esophageal cancers, including ESCC and EAC (28). Their system was trained using 8,428 endoscopic still images of esophageal cancer, including 365 ESCC cases and 32 EAC cases. It was then validated using a dataset consisting of 1,118 images from 97 cases (47 cases with esophageal cancer and 50 cases without it). The system accurately detected the cancer in 98% of the cases (46 of the 47), and analysed 1,118 images in 27 seconds. Notably, the CAD diagnostic system could detect all the lesions that were less than 10 mm in size. Moreover, it could specifically differentiate superficial and advanced cancer with 98% accuracy. The sensitivity of the system for each image was found to be 77%, with a specificity of 79%, positive predictive value (PPV) of 39%, and negative predictive value (NPV) of 95%. Although the PPV was not very high, the authors suggested that further training the system on normal structures and benign lesions would improve it. This was the first study to evaluate the possibility of detecting esophageal cancer in endoscopic images using a CAD system.
Ohmori et al. reported a CNN-based AI system to detect and differentiate ESCC using non-magnified endoscopy (non-ME) and ME images, respectively (29). They used 9,591 non-ME and 7,844 ME images of superficial ESCCs and 1,692 non-ME and 3,435 ME images of noncancerous lesions or normal esophagi to train the system. The validation dataset contained 255 non-ME WLI, 268 non-ME NBI/BLI, and 204 ME NBI/BLI from 135 patients. Fifteen experienced endoscopists diagnosed the same validation data. There was no significant difference in the diagnostic performance between the CAD system and the experienced endoscopists. The possibility of achieving detection and differentiation of ESCC in real-time using video images was suggested, because the CAD system performed its analysis at a rate of 36 images per second.
Cai et al. developed a deep neural network (DNN)-based CAD system trained using 2,428 (1,332 abnormal, 1,096 normal) images from 746 patients. A validation dataset consisting of 187 images from 52 patients was prepared to detect ESCC (30). The same validation data were diagnosed by 16 endoscopists (4 senior, 6 mid-level, and 6 junior). The DNN-based CAD system exhibited a diagnostic accuracy of 91.4%. Although the senior group exhibited an average diagnostic accuracy of 88.8%, the junior group exhibited a lower value of 77.2%. Interestingly, the sensitivity of endoscopists in detecting ESCC was improved in the image set that had a rectangular frame displayed by the CAD system to indicate ESCC. The average diagnostic ability of the endoscopists, after referring to the results of the CAD system, improved particularly in terms of sensitivity (74.2% vs. 89.2%), accuracy (81.7% vs. 91.1%), and NPV (79.3% vs. 90.4%). In terms of sensitivity and specificity, the CAD system was superior to both inexperienced and experienced endoscopists in detecting ESCC in endoscopic images.
Guo et al. trained a CAD system using 6,473 NBI still images (2,770 of precancerous lesions and early ESCC and 3,703 of noncancerous lesions) (31). To validate the system, 1,480 malignant and 5,191 noncancerous NBI still images as well as 20 ME and 27 non-ME NBI videos were used. The sensitivity and specificity of detecting ESCC in the still images were shown to be 98% and 95%, respectively, whereas those in video datasets were shown to be 100% in both non-ME and ME NBI. They concluded that the real-time CAD system has the potential to assist endoscopists in diagnosing precancerous lesions and ESCC in the near future.
Although the above mentioned reports have demonstrated highly accurate CAD systems using still endoscopic images, only one report has been validated with videos, in which the endoscopy is performed slowly (according to the video, it takes approximately 99 s from the esophagogastric junction to the cervical esophagus). In addition, the reports exclude poor quality images resulting from mucus, blur, halation, defocus, and poor insufflation of the air from validation videos, despite low-quality images being included alongside high-quality ones in clinical practice. Therefore, it can be concluded that these CAD systems require further improvement to detect early ESCC in a clinical setting, particularly in screening endoscopies, in which the endoscope is quickly passed through esophagus. Moreover, advancement in CNN detection still remains at the research stage. To address these weaknesses, it would be preferable to train the CAD system using endoscopic videos that include plenty of bridging images to achieve a higher robustness. Further, the system should be validated using high-speed videos in which the endoscope is passed from the esophagogastric junction to the cervical esophagus within approximately 10 s, as is the actual speed used in clinical practice. It would be useful to be able to predict high-risk cases of ESCC using standard WLI or NBI findings, similar to the prediction of cancer risks through iodine staining of Lugol-voiding lesions (LVL). It would also be useful if such a prediction could be made from a combination of endoscopic findings and clinical information, such as the amount of cigarette and alcohol consumption and blood test results.
Staging of ESCC
The risk of lymph node metastases is almost 0% for intraepithelial (EP) and lamina propria (LPM) ESCC, whereas it increases to 8–15%, 11–53%, and 30–54% for muscularis mucosa (MM), submucosal microinvasive (SM1), and submucosal deep invasive (SM2) or deeper cases, respectively (2-5). According to the Japan Esophageal Society (JES) guidelines, endoscopic resection (ER) is recommended for treating EP or LPM as definitive indication and MM or SM1 as relative indication, whereas surgical operation or chemoradiotherapy is recommended for SM2 cases (6). Thus, it is very important to diagnose the invasion depth of ESCC accurately to avoid over-treatment and improve patients’ quality of life. There are various diagnostic modalities to measure the invasion depth, such as regular WLI, endoscopic ultrasound (EUS), and ME with NBI (ME-NBI). ME-NBI enables improved visualization of subtle microvascular patterns in the esophageal mucosa of patients with ESCC (32). The JES classification (33,34), the Intrapapillary capillary loops (IPCL) classification of ESCC, is widely accepted. Importantly, each IPCL subgroup corresponds with high accuracy to histological findings and the invasion depth of esophageal squamous cell neoplasia (ESCN). However, it is challenging to diagnose invasion depth using WLI, ME-NBI, and EUS, especially for non-expert endoscopists (35,36). Therefore, a more advanced measurement approach is required to help non-experts achieve diagnosis quality equivalent to that of experts without using special techniques.
Tokai et al. reported a CNN-based CAD system to detect and subsequently diagnose the invasion depth of ESCC distinguishing EP-SM1 and depths below SM2 in superficial ESCC using non-magnifying WLI images (37) (Figure 1). They retrospectively collected 1,751 training images of early ESCC, and 291 images were prepared as a validation set to evaluate the diagnostic accuracy. These images were also reviewed by 13 board-certified endoscopists. The CAD system detected 95.5% (279/291) of the ESCC in the validation set. The overall accuracy of the obtained invasion depths of ESCC was 80.9% (225/279), whereas the corresponding sensitivity and specificity in diagnosing pEP-SM1 were 84.1% (159/189) and 73.3% (66/90), respectively. In this study, because they considered it crucial yet challenging to differentiate between MM-SM1 and SM2 cases to determine the treatment method, they prepared a higher number of MM-SM1 cases (41.9%). The diagnostic accuracy of this system exceeded that of 12 of the 13 expert endoscopists, and its score, measured by area under the curve, was greater than those of all the endoscopists.
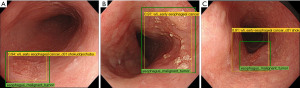
Nakagawa et al. also reported a positive outcome of a CAD system diagnosing the invasion depth of ESCC using both non-magnified and magnified images (38). They used 8,660 non-ME and 5,678 ME superficial ESCC images as a training dataset, and 405 non-ME images and 509 ME images as a validation set. The sensitivity, specificity, PPV, NPV, and accuracy for differentiating pathologic mucosal and SM1 cancers from SM2 or SM3 cancers were 90.1%, 95.8%, 99.2%, 63.9%, and 91.0%, respectively. The same validation data were diagnosed by 16 experienced endoscopists with overall sensitivity, specificity, PPV, NPV, and accuracy of 89.8%, 88.3%, 97.9%, 65.5%, and 89.6%, respectively.
Everson et al. reported the AI classification of intrapapillary capillary loop (IPCL) patterns for ESCC (39). A total of 7,046 sequential high-definition ME-NBI images from 17 patients (10 ESCN, 7 normal) were used to train a CAD system. This system could differentiate between abnormal and normal IPCL patterns in ME-NBI images with 93.7% accuracy.
Zhao et al. (40) produced a CAD system based on ME-NBI to investigate the classification of IPCLs automatically, and assist to diagnose early ESCC. The mean diagnostic accuracy of the model was 89.2%, which was significantly higher than that of the mid-level and junior doctor groups. This finding proved the feasibility of their model.
According to the abovementioned reports on invasion depth diagnosis, all the proposed systems showed good outcomes. However, certain concerns can be identified. First, the issue of selection bias in the validation set prevails. Nakagawa et al. (38) used several EP/LPM cases (75.5%), and fewer MM (6.5%) and SM1 cases (2.6%) in their validation set, and their system subsequently showed a high sensitivity of 90.1% in differentiating pathologic EP/SM1 cancers from SM2 cancers. On the other hand, Tokai et al. (37) used fewer EP/LPM cases (36%), and a higher number of MM/SM1 cases (40%) in their validation set, and their system showed a sensitivity of 84.1% in differentiating pathologic EP/SM1 cancers from SM2 cancers. Their study further evaluated the accuracy of CAD system for each pathological invasion depth; the accuracies of the EP-SM1 diagnoses were higher for the pEP-LPM and pMM groups (91.2% and 91.4%, respectively) than for the pSM1 group (67.8%). From these results, it can be said that although positive outcomes can be achieved easily using a large number of EP/LPM cases to distinguish them from EP-SM1 and SM2 cases, it is difficult to differentiate between the SM1 and SM2 cases. This weakness should be addressed before such systems are applied to clinical practice. Second, CAD systems trained using video data are necessary. This is because it is very important to observe the changes in tumor extension from the amount of insufflated air to understand the rigidity, flexibility, and thickness of the lesion while diagnosing the invasion depth; such findings can be observed in greater detail in videos as compared to still images. Third, it would be preferable if a CAD system could diagnose not only invasion depth but also the possibility of lymph node metastasis or lymphovascular invasion, location of deep invasion, location of ductal involvement, and existence of droplet infiltration. This would help in determining the management of the lesion as well as in performing endoscopic resection.
EAC in BE
It is well known that BE is a risk factor for the development of EAC. The risk of EAC rises when BE is associated with dysplasia (41,42), and its prognosis depends strongly on the stage at the time of diagnosis (43). Therefore, it is crucial to detect EAC in its early stage to improve the prognosis; however, the detection and characterization of early EAC in BE is challenging, especially for inexperienced endoscopists. Early EAC is difficult to detect from the surrounding non-dysplastic Barrett’s mucosa due to the flatness of the lesion, even with high-definition endoscopes (44).
It has been reported that high-definition white light endoscopy is the optimum solution for initial recognition of BE neoplasia by surveillance (45,46). The image quality of high-definition endoscopes is now sufficiently high for expert endoscopists to detect even very subtle mucosal surface abnormalities derived from high-grade dysplasia and early EAC (47). Despite these major improvements, detecting high-grade dysplasia and early EAC remains difficult for non-expert or general endoscopists who perform surveillance (47). This is because these endoscopists rarely encounter neoplastic lesions in BE (48). Therefore, another diagnostic modality is needed to assist non-expert or general endoscopists improve their accuracy of detecting early neoplasia in BE.
Detection of EAC
Ebigbo et al. used two databases, the Augsburg database and the Medical Image Computing and Computer Assisted-Intervention (MICCAI) database, to train and validate a CAD system driven by a CNN with a residual network (ResNet) architecture (49). The Augsburg dataset included 148 high-definition WLI and NBI images and 41 areas of non-neoplastic mucosa in BE, whereas the MICCAI dataset contained 100 high-definition WLI images of early EAC cases and 22 areas of non-neoplastic mucosa in BE. Using the Augsburg data, the sensitivity and specificity for diagnosing EAC are 97% and 88% for WLI, and 94% and 80% for NBI, respectively, which was superior to those of 11 of the 13 endoscopists. The sensitivity and specificity for the MICCAI images were 92% and 100%, respectively (49). Based on this work, Ebigbo et al. developed a real-time AI system (50). They trained the CAD system using a total of 129 endoscopic images. To validate in real time, additional images (36 early EAC and 26 normal BE) were assessed by the CAD system in concurrence with the endoscopic examination by an expert BE endoscopist. The CAD system showed sensitivity, specificity, and overall accuracy of 83.7%, 100.0%, and 89.9%, respectively.
Hashimoto et al. (51) reported a system that achieved not only accurate detection of early esophageal neoplasia in BE images, but also localization accuracy. They collected 916 images of high-grade dysplasia or T1 cancer in BE, and the lesion area was masked by image annotation software. They additionally collected 919 control images of BE without lesion. A CNN algorithm was pretrained on ImageNet and then fine-tuned with the goal of providing an accurate binary classification of “dysplastic” or “nondysplastic”. Then, an object detection algorithm that drew localization boxes around regions classified as dysplasia was developed. Concerning localization accuracy, they used mean average precision (mAP), usually used for AI. The CAD system analyzed 458 images (233 nondysplasia and 225) as a validation dataset and accurately detected early neoplasia with a sensitivity, specificity, and overall accuracy of 96.4%, 94.2%, and 95.4%, respectively. The localization algorithm accurately detected most lesions, with a mAP of 0.7533. In addition, the speed of predictions was greater than 70 fps. Hence, this work showed the possibility of developing a CAD system for detection of dysplasia for real-time endoscopy.
de Groof et al. (52) worked on developing a CAD system for detection of Barrett’s neoplasia. In this study, detection was considered to be accurate when the algorithm correctly identified an image as neoplastic or non-neoplastic. Furthermore, overlapped neoplastic areas delineated by experts and the CAD system were evaluated. Prospective collection of WLI for suspected areas of Barrett’s from 40 Barrett’s and 20 non-dysplastic patients was delineated by six expert endoscopists. The system achieved accuracy, sensitivity, and specificity of 91.7%, 95%, and 85%, respectively per image; the lesion was successfully located. The algorithm rapidly identified BE, with a mean time of 1.051 seconds per image using WLI. They also developed a hybrid ResNet-UNet model CAD system using five well-defined, independent endoscopy datasets (53) to train and validate it. Pre-training was performed using 494,364 labelled endoscopic images collected from all intestinal segments. Then, a total of 1,704 images of early BE neoplasia and nondysplastic BE, derived from four different centers and delineated by >10 experts, were acquired for training and validation. System performance was assessed using the fourth and fifth datasets. The fifth dataset was also scored by 53 general endoscopists from four different countries. The system differentiated images containing neoplasms or nondysplastic BE with accuracy, sensitivity, and specificity of 89%, 90%, and 88%, respectively (fourth dataset, 80 patients and images) and 88%, 93%, and 83%, respectively (fifth dataset, 80 patients and images). Meanwhile, the general endoscopists achieved accuracy, sensitivity, and specificity of 73%, 72%, and 74%, respectively. The CAD system showed higher accuracy than 53 inexpert endoscopists, with comparable delineation performance. The CAD system identified the optimal site for the biopsy of detected neoplasia in 97% and 92% of cases (the fourth and fifth datasets, respectively).
It is notable that the CAD system can identify the area suitable for biopsy (52), because it is difficult to accurately determine the biopsy location of early EAC in BE, for both non-experienced and experienced endoscopists. Such solutions would overcome the issue of random biopsy. Although the reports showed good outcomes in detecting EAC, we believe that video analysis is required for both training and validation to achieve improved clinical usability. Anatomically, it is highly challenging to observe the esophageal mucosa near esophagogastric junction, particularly for non-experts. Therefore, a CAD system using real-time video that can detect EAC under difficult conditions, such as at the esophagogastric junction, is needed.
Conclusions
AI-based research focused on esophageal cancers is increasing, particularly in the detection and staging of ESCC and EAC. We hope further development of CAD systems will be applied in clinical practice, such as real-time video-based systems and ESCC risk prediction systems based on the images of non-cancerous esophagus. With these achievements, AI systems can effectively assist endoscopists in making accurate diagnoses and could increase the rate of detection of esophageal cancers at an early stage, improving survival rates. Further, improved diagnoses of invasion depth would improve patients’ quality of life by avoiding over-treatment.
Acknowledgments
Funding: None.
Footnote
Provenance and Peer Review: This article was commissioned by the Guest Editors (Hon Chi Yip and Philip Wai-Yan Chiu) for the series “Endoscopic Diagnosis and Treatment of Early Esophageal Cancer” published in Annals of Esophagus. The article has undergone external peer review.
Conflicts of Interest: All authors have completed the ICMJE uniform disclosure form (available at https://aoe.amegroups.com/article/view/10.21037/aoe-2020-33/coif). The series “Endoscopic Diagnosis and Treatment of Early Esophageal Cancer” was commissioned by the editorial office without any funding or sponsorship. The authors have no other conflicts of interest to declare.
Ethical Statement: The authors are accountable for all aspects of the work in ensuring that questions related to the accuracy or integrity of any part of the work are appropriately investigated and resolved.
Open Access Statement: This is an Open Access article distributed in accordance with the Creative Commons Attribution-NonCommercial-NoDerivs 4.0 International License (CC BY-NC-ND 4.0), which permits the non-commercial replication and distribution of the article with the strict proviso that no changes or edits are made and the original work is properly cited (including links to both the formal publication through the relevant DOI and the license). See: https://creativecommons.org/licenses/by-nc-nd/4.0/.
References
- Klang E. Deep learning and medical imaging. J Thorac Dis 2018;10:1325-8. [Crossref] [PubMed]
- Chartrand G, Cheng PM, Vorontsov E, et al. Deep learning: a primer for radiologists. Radiographics 2017;37:2113-31. [Crossref] [PubMed]
- Litjens G, Kooi T, Bejnordi BE, et al. A survey on deep learning in medical image analysis. Med Image Anal 2017;42:60-88. [Crossref] [PubMed]
- Watson DS, Krutzinna J, Bruce IN, et al. Clinical applications of machine learning algorithms: beyond the black box. BMJ 2019;364:l886. [Crossref] [PubMed]
- Barash Y, Klang E. Automated quantitative assessment of oncological disease progression using deep learning. Ann Transl Med 2019;7:S379. [Crossref] [PubMed]
- Wang P, Xiao X, Glissen Brown JR, et al. Development and validation of a deep-learning algorithm for the detection of polyps during colonoscopy. Nat Biomed Eng 2018;2:741-8. [Crossref] [PubMed]
- Menon S, Trudgill N. How commonly is upper gastrointestinal cancer missed at endoscopy? A meta-analysis. Endosc Int Open 2014;2:E46-50. [Crossref] [PubMed]
- Rodríguez de Santiago E, Hernanz N, Marcos-Prieto HM, et al. Rate of missed oesophageal cancer at routine endoscopy and survival outcomes: a multicentric cohort study. United European Gastroenterol J 2019;7:189-98. [Crossref] [PubMed]
- Kamangar F, Dores GM, Anderson WF. Patterns of cancer incidence, mortality, and prevalence across five continents: defining priorities to reduce cancer disparities in different geographic regions of the world. J Clin Oncol 2006;24:2137-50. [Crossref] [PubMed]
- Wei WQ, Chen ZF, He YT, et al. Long-term follow-up of a community assignment, one-time endoscopic screening study of esophageal cancer in China. J Clin Oncol 2015;33:1951-57. [Crossref] [PubMed]
- Ferlay J, Colombet M, Soerjomataram I, et al. Estimating the global cancer incidence and mortality in 2018:GLOBOCAN sources and methods. Int J Cancer 2019;144:1941-53. [Crossref] [PubMed]
- Kodama M, Kakegawa T. Treatment of superficial cancer of the esophagus: a summary of responses to a questionnaire on superficial cancer of the esophagus in Japan. Surgery 1998;123:432-9.
- Yamashina T, Ishihara R, Nagai K, et al. Long-term outcome and metastatic risk after endoscopic resection of superficial esophageal squamous cell carcinoma. Am J Gastroenterol 2013;108:544-51. [Crossref] [PubMed]
- Katada C, Muto M, Momma K, et al. Clinical outcome after endoscopic mucosal resection for esophageal squamous cell carcinoma invading the muscularis mucosae-a multicenter retrospective cohort study. Endoscopy 2007;39:779-83. [Crossref] [PubMed]
- Shimizu Y, Tsukagoshi H, Fujita M, et al. Long-term outcome after endoscopic mucosal resection in patients with esophageal squamous cell carcinoma invading the muscularis mucosae or deeper. Gastrointest Endosc 2002;56:387-90. [Crossref] [PubMed]
- Igaki H, Kato H, Tachimori Y, et al. Clinicopathologic characteristics and survival of patients with clinical stage I squamous cell carcinomas of the thoracic esophagus treated with three-field lymph node dissection. Eur J Cardiothorac Surg 2001;20:1089-94. [Crossref] [PubMed]
- Yamamoto S, Ishihara R, Motoori M, et al. Comparison between definitive chemoradiotherapy and esophagectomy in patients with clinical stage I esophageal squamous cell carcinoma. Am J Gastroenterol 2011;106:1048-54. [Crossref] [PubMed]
- Hashimoto CL, Iriya K, Baba ER, et al. Lugol’s dye spray chromoendoscopy establishes early diagnosis of esophageal cancer in patients with primary head and neck cancer. Am J Gastroenterol 2005;100:275-82. [Crossref] [PubMed]
- Dawsey SM, Fleischer DE, Wang GQ, et al. Mucosal iodine staining improves endoscopic visualization of squamous dysplasia and squamous cell carcinoma of the esophagus in Linxian, China. Cancer 1998;83:220-31.
- Muto M, Minashi K, Yano T, et al. Early detection of superficial squamous cell carcinoma in the head and neck region and esophagus by narrow band imaging: a multicenter randomized controlled trial. J Clin Oncol 2010;28:1566-72. [Crossref] [PubMed]
- Nagami Y, Tominaga K, Machida H, et al. Usefulness of non-magnifying narrow-band imaging in screening of early esophageal squamous cell carcinoma: a prospective comparative study using propensity score matching. Am J Gastroenterol 2014;109:845-54. [Crossref] [PubMed]
- Lee YC, Wang CP, Chen CC, et al. Transnasal endoscopy with narrowband imaging and Lugol staining to screen patients with head and neck cancer whose condition limits oral intubation with standard endoscope (with video). Gastrointest Endosc 2009;69:408-17. [Crossref] [PubMed]
- Kuraoka K, Hoshino E, Tsuchida T, et al. Early esophageal cancer can be detected by screening endoscopy assisted with narrow-band imaging (NBI). Hepatogastroenterology 2009;56:63-6.
- Kaneko K, Oono Y, Yano T, et al. Effect of novel bright image enhanced endoscopy using blue laser imaging (BLI). Endosc Int Open 2014;2:E212-9. [Crossref] [PubMed]
- Tomie A, Dohi O, Yagi N, et al. Blue laser imaging-bright improves endoscopic recognition of superficial esophageal squamous cell carcinoma. Gastroenterol Res Pract 2016;2016:6140854. [Crossref] [PubMed]
- Morita FH, Bernardo WM, Ide E, et al. Narrow band imaging versus Lugol chromoendoscopy to diagnose squamous cell carcinoma of the esophagus: a systematic review and meta-analysis. BMC Cancer 2017;17:54. [Crossref] [PubMed]
- Ishihara R, Takeuchi Y, Chatani R, et al. Prospective evaluation of narrow-band imaging endoscopy for screening of esophageal squamous mucosal high-grade neoplasia in experienced and less experienced endoscopists. Dis Esophagus 2010;23:480-6. [Crossref] [PubMed]
- Horie Y, Yoshio T, Aoyama K, et al. Diagnostic outcomes of esophageal cancer by artificial intelligence using convolutional neural networks. Gastrointest Endosc 2019;89:25-32. [Crossref] [PubMed]
- Ohmori M, Ishihara R, Aoyama K, et al. Endoscopic detection and differentiation of esophageal lesions using a deep neural network. Gastrointest Endosc 2020;91:301-9.e1. [Crossref] [PubMed]
- Cai SL, Li B, Tan WM, et al. Using a deep learning system in endoscopy for screening of early esophageal squamous cell carcinoma (with video). Gastrointest Endosc 2019;90:745-53.e2. [Crossref] [PubMed]
- Guo L, Xiao X, Wu C, et al. Real-time automated diagnosis of precancerous lesions and early esophageal squamous cell carcinoma using a deep learning model (with videos). Gastrointest Endosc 2020;91:41-51. [Crossref] [PubMed]
- Gono K, Obi T, Yamaguchi M, et al. Appearance of enhanced tissue features in narrow-band endoscopic imaging. J Biomed Opt 2004;9:568-77. [Crossref] [PubMed]
- Oyama T, Inoue H, Arima M, et al. Prediction of the invasion depth of superficial squamous cell carcinoma based on microvessel morphology: Magnifying endoscopic classification of the Japan Esophageal Society. Esophagus 2017;14:105-12. [Crossref] [PubMed]
- Oyama T, Momma K. A new classification of magnified endoscopy for superficial esophageal squamous cell carcinoma. Esophagus 2011;8:247-51.
- Ebi M, Shimura T, Yamada T, et al. Multicenter, prospective trial of white-light imaging alone versus white-light imaging followed by magnifying endoscopy with narrow-band imaging for the real-time imaging and diagnosis of invasion depth in superficial esophageal squamous cell carcinoma. Gastrointest Endosc 2015;81:1355-61.e2. [Crossref] [PubMed]
- Thosani N, Singh H, Kapadia A, et al. Diagnostic accuracy of EUS in differentiating mucosal versus submucosal invasion of superficial esophageal cancers: a systematic review and meta-analysis. Gastrointest Endosc 2012;75:242-53. [Crossref] [PubMed]
- Tokai Y, Yoshio T, Aoyama K, et al. Application of artificial intelligence using convolutional neural networks in determining the invasion depth of esophageal squamous cell carcinoma. Esophagus 2020;17:250-6. [Crossref] [PubMed]
- Nakagawa K, Ishihara R, Aoyama K, et al. Classification for invasion depth of esophageal squamous cell carcinoma using a deep neural network compared with experienced endoscopists. Gastrointest Endosc 2019;90:407-14. [Crossref] [PubMed]
- Everson M, Herrera LCGP, Li W, et al. Artificial intelligence for the real-time classification of intrapapillary capillary loop patterns in the endoscopic diagnosis of early oesophageal squamous cell carcinoma: A proof-of-concept study. United European Gastroenterol J 2019;7:297-306. [Crossref] [PubMed]
- Zhao YY, Xue DX, Wang YL, et al. Computer-assisted diagnosis of early esophageal squamous cell carcinoma using narrow-band imaging magnifying endoscopy. Endoscopy 2019;51:333-41. [Crossref] [PubMed]
- Curvers WL, Ten Kate FJ, Krishnadath KK, et al. Low-grade dysplasia in Barrett’s esophagus: overdiagnosed and underestimated. Am J Gastroenterol 2010;105:1523-30. [Crossref] [PubMed]
- Rastogi A, Puli S, El-Serag HB, et al. Incidence of esophageal adenocarcinoma in patients with Barrett’s esophagus and highgrade dysplasia: a meta-analysis. Gastrointest Endosc 2008;67:394-8. [Crossref] [PubMed]
- Hur C, Miller M, Kong CY, et al. Trends in esophageal adenocarcinoma incidence and mortality. Cancer 2013;119:1149-58. [Crossref] [PubMed]
- Davis-Yadley AH, Neill KG, Malafa MP, et al. Advances in the endoscopic diagnosis of Barrett esophagus. Cancer Control 2016;23:67-77. [Crossref] [PubMed]
- Spechler SJ, Sharma P, Souza RF, et al. American Gastroenterological Association medical position statement on the management of Barrett’s esophagus. Gastroenterology 2011;140:1084-91. [Crossref] [PubMed]
- Weusten B, Bisschops R, Coron E, et al. Endoscopic management of Barrett’s esophagus: European Society of Gastrointestinal Endoscopy (ESGE) position statement. Endoscopy 2017;49:191-8. [Crossref] [PubMed]
- Schölvinck DW, van der Meulen K, Bergman JJ, et al. Detection of lesions in dysplastic Barrett’s esophagus by community and expert endoscopists. Endoscopy 2017;49:113-20. [Crossref] [PubMed]
- Hvid-Jensen F, Pedersen L, Drewes AM, et al. Incidence of adenocarcinoma among patients with Barrett’s esophagus. N Engl J Med 2011;365:1375-83. [Crossref] [PubMed]
- Ebigbo A, Mendel R, Probst A, et al. Computer-aided diagnosis using deep learning in the evaluation of early oesophageal adenocarcinoma. Gut 2019;68:1143-5. [Crossref] [PubMed]
- Ebigbo A, Mendel R, Probst A, et al. Real-time use of artificial intelligence in the evaluation of cancer in Barrett’s oesophagus. Gut 2020;69:615-6. [Crossref] [PubMed]
- Hashimoto R, Requa J, Tyler D, et al. Artificial intelligence using convolutional neural networks for real-time detection of early esophageal neoplasia in Barrett's esophagus (with video). Gastrointest Endosc 2020;91:1264-1271.e1. [Crossref] [PubMed]
- de Groof J, van der Sommen F, van der Putten J, et al. The Argos project: the development of a computer-aided detection system to improve detection of Barrett’s neoplasia on white light endoscopy. United European Gastroenterol J 2019;7:538-47. [Crossref] [PubMed]
- de Groof Albert J, Struyvenberg Maarten R, van der Putten Joost, et al. Deep-learning system detects neoplasia in patients with Barrett’s esophagus with higher accuracy than endoscopists in a multistep training and validation study with benchmarking. Gastroenterology 2020;158:915-29.e4. [Crossref] [PubMed]
Cite this article as: Tokai Y, Yoshio T, Fujisaki J. Development of artificial intelligence for the detection and staging of esophageal cancer. Ann Esophagus 2023;6:3.